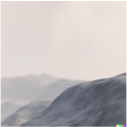


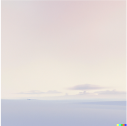
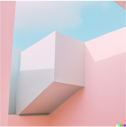


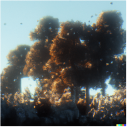



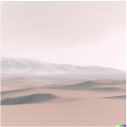

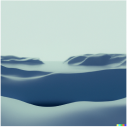


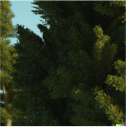



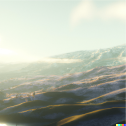
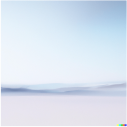

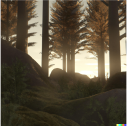

At Nymark we support tech-based change for good. This is our Reflections series, where we take a step back to look at the industry’s big issues. With a humble curiosity for not just what is being done, but how. To better understand the red threads through industries, and uncover shared challenges and solutions for technologies that make a difference. Here we consider what ethical AI means in the context of sustainability, in an effort to unpack the steps toward sustainable AI and process for organisations today.
The emergence of new AI tools are redefining ways of working. Whether its policy makers, business leaders, or anyone looking to make the most of ‘daily-life’ AI tools, broadly, we are striving to speak the same language around AI use. Before it becomes a barrier to solving complex AI problems. Still, we are seeking alignment around some basic norms. Such as, what is it ok to use AI for, and how? What does it mean to use AI for good? How can we build sustainable approaches to AI use?
AI’s impact on sustainability, and its potential to help us fight the climate crisis, is unclear too. There is promise in AI built for sustainability: environmental and climate technologies using AI as a tool to address environmental and social challenges. Research suggests that AI-powered solutions could mitigate 5 to 10% of global greenhouse gas emissions by 2030. AI is also expected to accelerate the emergence and impact of climate technologies, such as nuclear fusion, and streamline analysis of emissions through better tracking and data.
Separate to this is Sustainable AI: efforts toward the responsible development and operation of AI technologies to minimise the environmental impact of AI systems. Today, there are fears surrounding AI’s as-yet unknown impact on the climate and a lack of clarity around its energy use. The shift in technology could already be amassing huge amounts of computational energy, currently unaccounted for; a lack of transparency from AI companies further obscures our understanding of the impact on emissions from today’s models. Research says US power plants could be prolonged to meet the rising energy demands of AI, while AI servers might soon demand the energy consumption of Sweden.
We can expect to hear more about Sustainable AI. Green AI pipelines require more education on sustainable software engineering and knowledge around hardware use, such as identifying a GPU appropriate to an organisation’s needs. This conversation is just beginning. Even sustainable software scholars from TU Delft say more work is needed to bridge these gaps in their knowledge.
Another argument from green AI experts suggests moving away from the prevalent Big Data mindset towards a Small Data approach. Reducing the energy consumption of AI through techniques such as simplification – a data selection strategy that only collects data that matters for the model and application at hand – and distillation, the transfer of knowledge from larger models into smaller ones.
At the core, ensuring AI is done right means redrawing its foundational issues. Not only complying with laws, or building AI with sustainability in mind, but a 360 view: the goal is to have AI that is legal, ethical, and robust. (EU regulation for ethical AI defines guidelines for trustworthy AI as ‘lawful: respecting all applicable laws and regulations, ethical: respecting ethical principles and values, and robust: both from a technical perspective while taking into account its social environment’.)
When these foundations aren’t in place, we have seen the consequences: AI hallucinates, it discriminates, it’s inaccurate. And without clear policy today, technology leaders must rise to the challenge of deploying ethical AI. By grounding approaches to AI through designing useful and accessible ethical frameworks.
Frameworks such as ethical AI principles can help build towards ethical and Sustainable AI by design. To reduce harm at a foundational level. And address the challenge of tech moving too fast for regulation to keep up. Ethical principles for AI are foundational principles that often come before the technology is built, defining ways of reducing harm such as bias and misinformation. For Sustainable AI, this means part of the value system should be to avoid climate harm, as well as building AI systems to help reduce it.
A leading framework for ethical AI principles is UNESCO’s Recommendation on the Ethics of Artificial Intelligence. It outlines special requirements considering AI’s implications in scientific knowledge, civic participation and decision making, among others. Values for Sustainable AI use here include ‘environmental and ecosystem flourishing promoted through the life cycle of AI systems’ and to ‘reduce the environmental impact of AI systems, including but not limited to its carbon footprint’.
Yet UNESCO’s framework also reminds us of the complexity of Sustainable AI. Through its Sustainability principle, dependent on: ‘a continuum of human, social, cultural, economic and environmental dimensions’ and assessment of AI’s implications against ‘a set of constantly evolving goals across a range of dimensions,’ such as the UN’s Sustainable Development Goals.
For compliance in high-risk, high-reward technologies, from climate to health tech, there are calls for AI regulation to focus on use case, over technology. High risk AI technologies could include, as an example, innovation in autonomous vehicles, against lower risk use cases, such as AI automation in business technology.
The EU outlines their approach to regulation per use case: a ‘proportionate risk-based approach’ alongside ‘codes of conduct for non-high-risk AI systems’. This route aims to support innovation – protecting safety or rights of citizens in higher-risk cases, while allowing organisations to harness the potential made possible by lower-risk AI tools. This means more mandatory supervision, transparency and documentation for high-risk systems, to ensure the characteristics of these use cases are considered to align with the EU’s Charter of Fundamental Rights. Including Article 37: the right to a high level of environmental protection and the improvement of the quality of the environment.
Ultimately, the ethical use of AI demands a lot of creative problem solving. And renewed, consistent processes to ensure we are building AI we can trust. This involves drawing up those foundational principles through which to judge their use of the technology against. To first ensure everyone speaks the same language, and is aligned on the values driving the use of AI.
Then, designing for AI use cases requires useful types of problem solving to understand challenges. Such as how the accuracy, safety, and transparency of the AI systems used in those contexts are particularly important. As an example here, systems thinking can be used to state assumptions, mitigate biases, help uncover overlooked issues and cross-dependencies. This leads to issue framing – modulating the size of the concern in order to solve it in a way that’s feasible and helpful.
When such foundations are in place, actioning them is an ongoing process of understanding how to apply them in day to day work. Training methods can educate and instil a trustworthy culture. And ethical AI also means recourse when something goes wrong. But who is really responsible is another sticky issue. For now, the head of AI role is still taking shape, and may be more of a figurehead.
Such as arguments being made by the likes of the EU's European AI Alliance, that “screening and assessing for unintended ethical and societal risk in AI applications should be introduced as a new requirement for CSR and ESG reporting.” With AI’s full impact on the environment and the workplace continually evolving, organisations might soon be tasked with redrawing approaches to risk assessments, to get ready for a new era of accountability. Proving that, for AI to strengthen our defences against the world’s biggest problems, it first requires us to strengthen our humanity, and ways of working together, in unique ways.